Recommendation Engine for Retail Coupons
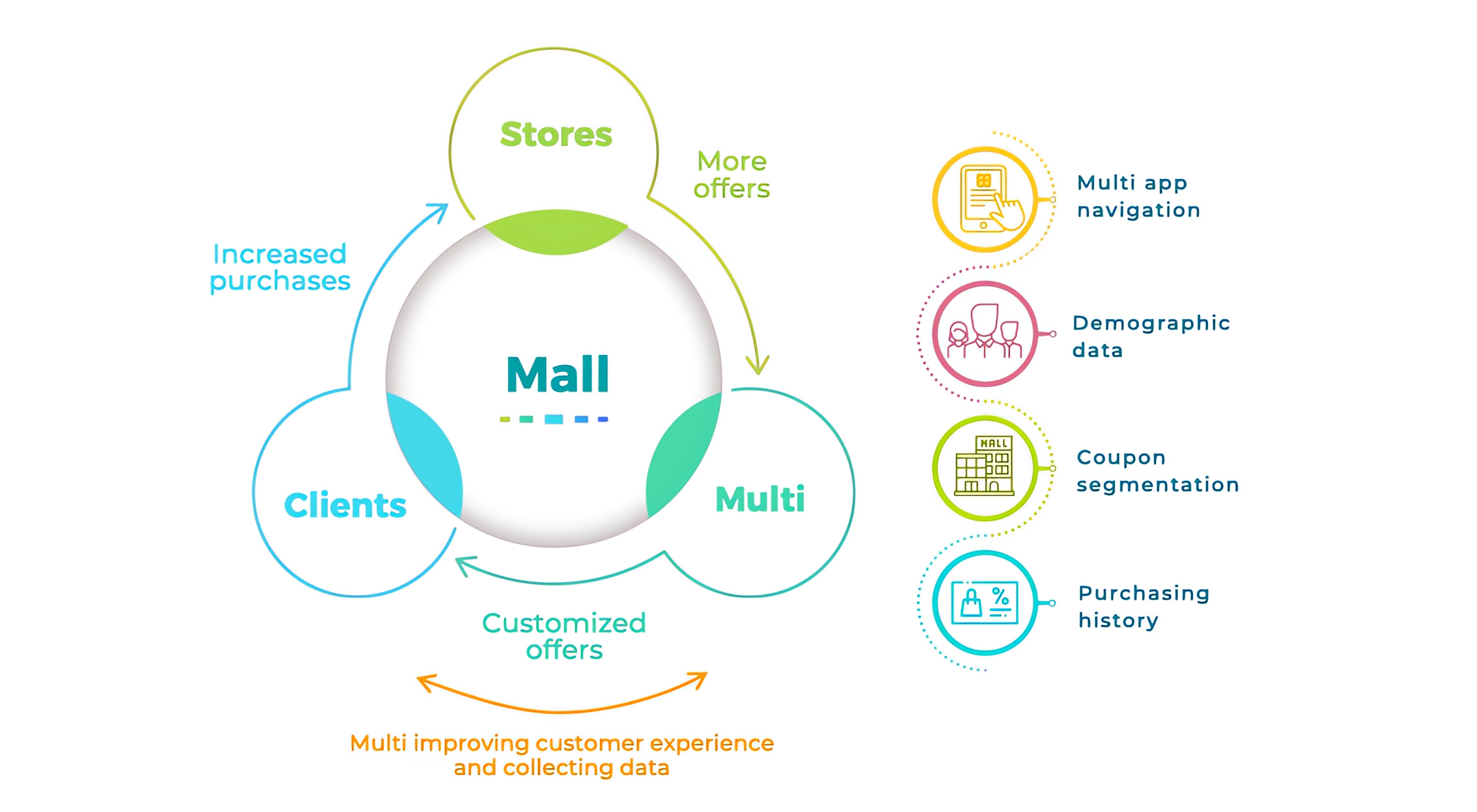
To boost app usage and increase coupon redemptions in a shopping mall super app, I developed a recommendation engine that delivers the most relevant coupons to users based on their individual preferences. The aim was to enhance customer engagement by offering personalized deals and drive more traffic and sales for mall tenants.
By integrating both online and offline customer data, such as browsing history in the app, previously activated coupons, demographics, and shopping preferences, I built a machine learning algorithm that analyzed this data to predict what each customer would be most interested in. The model considered combinations from more than 200 available offers across categories like leisure, gastronomy, fashion, cosmetics, and services. We introduced a dedicated personalized coupon window on the app's home screen, showing each user the offers most relevant to them, while activating users through personalized push notifications as well.
After the deployment of the model, we saw a 50% increase in coupon views and doubled the click-through rates within the first few weeks. Participating tenants experienced a 50% boost in customer traffic, with tens of thousands of coupon redemptions and additional mall visits. By sending targeted and relevant communications, we also improved message open rates by over 100% compared to the previous quarter.
You can read more about this analysis in the Investor Relations report this project was showcased in the Earnings Release 3Q21 (p. 14), 4Q21 (p. 20), 3Q22 (p. 10), 4Q22 (p. 12) [direct link to the PDF].